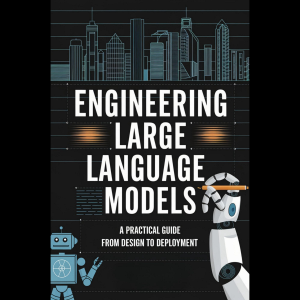
Engineering Large Language Models: A Practical Guide from Design to Deployment
Sanford Edwards
This audiobook is narrated by a digital voice.
Discover the world of large language models with this comprehensive guide, designed to take you from the initial design stages to the final deployment. This book provides a practical approach to understanding the complexities and intricacies involved in engineering these powerful AI systems. Whether you are a seasoned professional or a curious beginner, this guide offers valuable insights and hands-on knowledge to help you navigate the challenges and opportunities in this cutting-edge field. The book begins by exploring the foundational concepts and principles that underpin large language models. You will learn about the different architectures, algorithms, and techniques used to create these models, as well as the various tools and frameworks available to support your work. Each chapter builds on the previous one, providing a structured and cohesive learning experience that ensures you gain a deep understanding of the subject matter. As you progress through the book, you will encounter real-world examples and case studies that illustrate the practical applications of large language models. These examples cover a wide range of industries and use cases, from natural language processing and sentiment analysis to machine translation and text generation.
Duration - 7h 12m.
Author - Sanford Edwards.
Narrator - Digital Voice Michelle G.
Published Date - Wednesday, 15 January 2025.
Copyright - © 2025 James Kliewer ©.
Location:
United States
Description:
This audiobook is narrated by a digital voice. Discover the world of large language models with this comprehensive guide, designed to take you from the initial design stages to the final deployment. This book provides a practical approach to understanding the complexities and intricacies involved in engineering these powerful AI systems. Whether you are a seasoned professional or a curious beginner, this guide offers valuable insights and hands-on knowledge to help you navigate the challenges and opportunities in this cutting-edge field. The book begins by exploring the foundational concepts and principles that underpin large language models. You will learn about the different architectures, algorithms, and techniques used to create these models, as well as the various tools and frameworks available to support your work. Each chapter builds on the previous one, providing a structured and cohesive learning experience that ensures you gain a deep understanding of the subject matter. As you progress through the book, you will encounter real-world examples and case studies that illustrate the practical applications of large language models. These examples cover a wide range of industries and use cases, from natural language processing and sentiment analysis to machine translation and text generation. Duration - 7h 12m. Author - Sanford Edwards. Narrator - Digital Voice Michelle G. Published Date - Wednesday, 15 January 2025. Copyright - © 2025 James Kliewer ©.
Language:
English
Chapter 1: Introduction to Large Language Models 4
Duration:00:00:05
1.1 What are Large Language Models? 4
Duration:00:07:04
1.2 History and Evolution 9
Duration:00:06:12
1.3 Importance in Modern Technology 13
Duration:00:07:23
1.4 Overview of the Book 18
Duration:00:05:34
Chapter 2: Understanding the Basics of NLP 23
Duration:00:00:05
2.1 Natural Language Processing (NLP) Fundamentals 23
Duration:00:09:07
2.2 Key Concepts and Terminologies 29
Duration:00:10:40
2.3 Text Preprocessing Techniques 37
Duration:00:07:51
2.4 Text Representation Methods 42
Duration:00:05:59
Chapter 3: Fundamentals of Machine Learning for NLP 47
Duration:00:00:05
3.1 Supervised and Unsupervised Learning 47
Duration:00:06:44
3.2 Common Algorithms and Models 52
Duration:00:09:23
3.3 Evaluation Metrics for NLP Models 59
Duration:00:09:30
3.4 Handling Imbalanced Data 65
Duration:00:09:19
Chapter 4: Deep Learning for Large Language Models 73
Duration:00:00:05
4.1 Introduction to Deep Learning 73
Duration:00:07:30
4.2 Neural Networks and Architectures 78
Duration:00:06:14
4.3 Training Deep Learning Models 83
Duration:00:06:07
4.4 Regularization and Optimization Techniques 87
Duration:00:05:53
Chapter 5: Designing Large Language Models 92
Duration:00:00:04
5.1 Architecture Selection 92
Duration:00:07:57
5.2 Model Size and Complexity 98
Duration:00:05:22
5.3 Data Requirements and Collection 101
Duration:00:06:55
5.4 Ethical Considerations in Design 106
Duration:00:08:20
Chapter 6: Data Preparation and Management 113
Duration:00:00:05
6.1 Data Collection Strategies 113
Duration:00:07:54
6.2 Data Cleaning and Preprocessing 118
Duration:00:07:53
6.3 Data Augmentation Techniques 124
Duration:00:07:45
6.4 Managing Large-Scale Datasets 129
Duration:00:05:16
Chapter 7: Training Large Language Models 134
Duration:00:00:04
7.1 Training Process Overview 134
Duration:00:07:37
7.2 Distributed Training Techniques 139
Duration:00:06:11
7.3 Hardware and Software Requirements 144
Duration:00:07:23
7.4 Monitoring and Debugging Training Processes 149
Duration:00:08:34
Chapter 8: Fine-Tuning and Transfer Learning 156
Duration:00:00:04
8.1 Fine-Tuning Pre-Trained Models 156
Duration:00:07:45
8.2 Transfer Learning Techniques 161
Duration:00:06:37
8.3 Domain Adaptation 166
Duration:00:06:26
8.4 Case Studies and Best Practices 171
Duration:00:25:09
Chapter 9: Evaluation and Validation 189
Duration:00:00:05
9.1 Model Evaluation Metrics 189
Duration:00:07:06
9.2 Validation Techniques 194
Duration:00:07:13
9.3 Error Analysis 199
Duration:00:07:15
9.4 Improving Model Performance 204
Duration:00:06:24
Chapter 10: Deployment Strategies 209
Duration:00:00:04
10.1 Deployment Environments 209
Duration:00:06:16
10.2 Model Serving and Scaling 213
Duration:00:07:20
10.3 API Design and Integration 219
Duration:00:07:11
10.4 Monitoring and Maintenance 223
Duration:00:05:04
Chapter 11: Optimization and Performance Tuning 228
Duration:00:00:05
11.1 Model Compression Techniques 228
Duration:00:05:13
11.2 Quantization and Pruning 232
Duration:00:05:26
11.3 Efficient Inference Techniques 236
Duration:00:07:22
11.4 Performance Benchmarking 241
Duration:00:06:31
Chapter 12: Ethical and Societal Implications 246
Duration:00:00:05
12.1 Bias and Fairness in AI 246
Duration:00:07:31
12.2 Privacy Concerns 251
Duration:00:05:40
12.3 Transparency and Explainability 255
Duration:00:08:37
12.4 Regulatory and Compliance Issues 261
Duration:00:07:28
Chapter 13: Case Studies and Applications 267
Duration:00:00:04
13.1 Real-World Applications 267
Duration:00:07:39
13.2 Success Stories and Lessons Learned 272
Duration:00:06:23
13.3 Industry-Specific Use Cases 277
Duration:00:21:15
13.4 Future Trends and Innovations 291
Duration:00:07:19
Chapter 14: Conclusion and Future Directions 297
Duration:00:00:05
14.1 Recap of Key Concepts 297
Duration:00:04:47
14.2 Emerging Trends in NLP and AI 300
Duration:00:07:42
14.3 The Road Ahead for Large Language Models 306
Duration:00:08:29
14.4 Final Thoughts 311
Duration:00:07:01